출처 : https://spark.apache.org/docs/latest/structured-streaming-programming-guide.html#output-modes
Programming Model
The key idea in Structured Streaming is to treat a live data stream as a table that is being continuously appended. This leads to a new stream processing model that is very similar to a batch processing model. You will express your streaming computation as standard batch-like query as on a static table, and Spark runs it as an incremental query on the unbounded input table. Let’s understand this model in more detail.
> Struct Streaming 의 idea 는 연속된 append 구조이다. 너는 스트리밍 계산을 노말한 batch query 형태로 계산 할 수 있을 것이다.
Basic Concepts
Consider the input data stream as the “Input Table”. Every data item that is arriving on the stream is like a new row being appended to the Input Table.
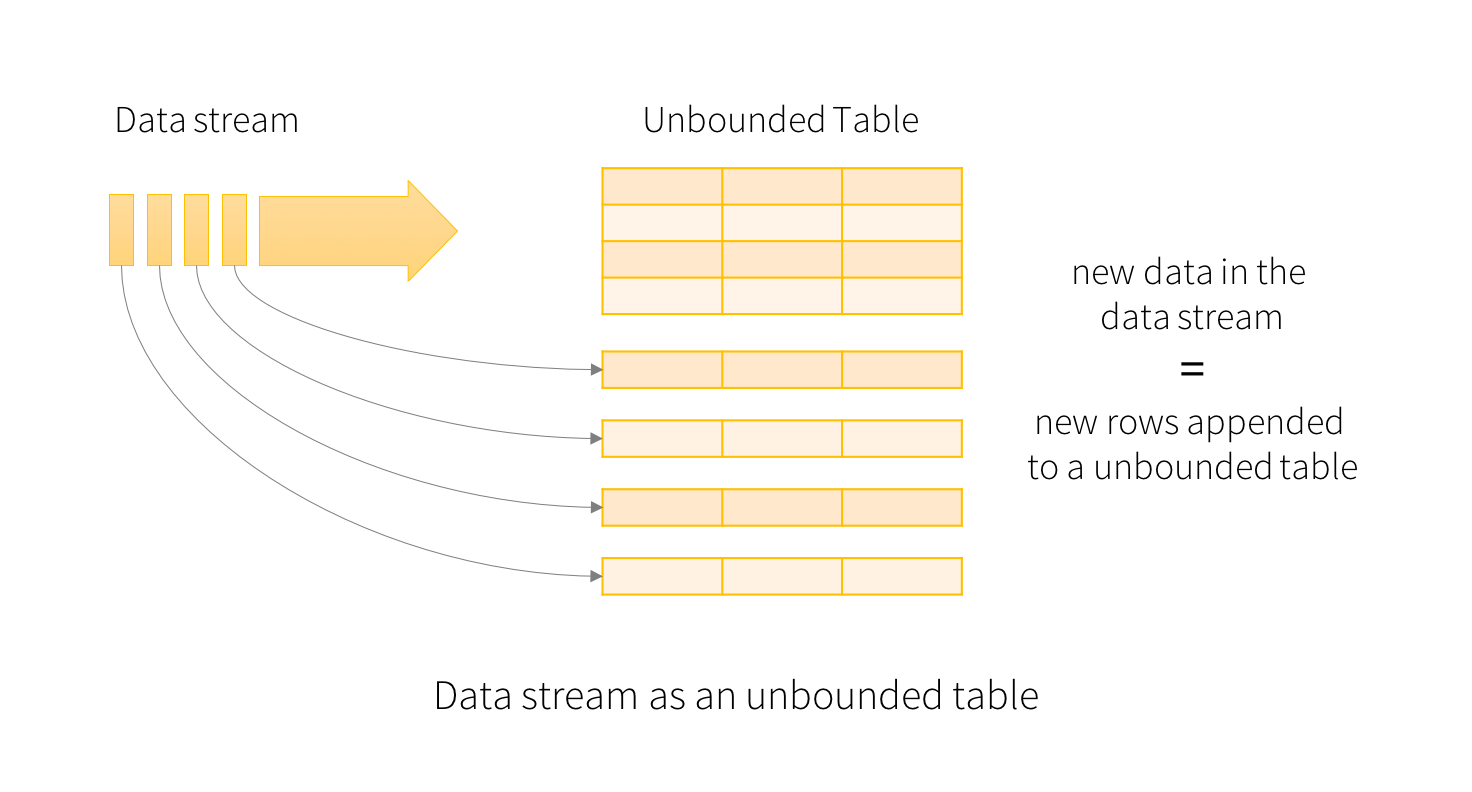
A query on the input will generate the “Result Table”. Every trigger interval (say, every 1 second), new rows get appended to the Input Table, which eventually updates the Result Table. Whenever the result table gets updated, we would want to write the changed result rows to an external sink.
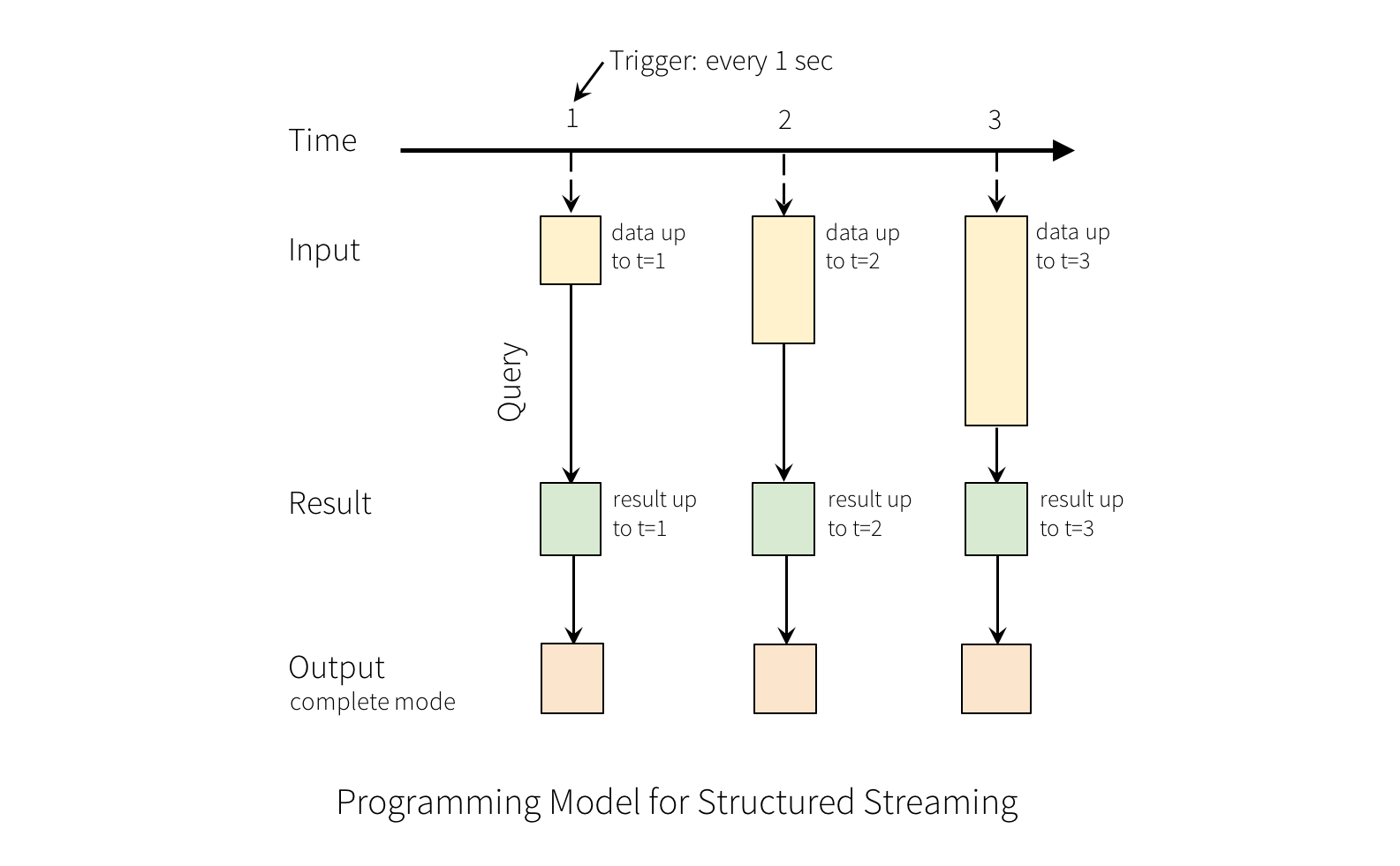
The “Output” is defined as what gets written out to the external storage. The output can be defined in a different mode:
Complete Mode - The entire updated Result Table will be written to the external storage. It is up to the storage connector to decide how to handle writing of the entire table.
Append Mode - Only the new rows appended in the Result Table since the last trigger will be written to the external storage. This is applicable only on the queries where existing rows in the Result Table are not expected to change.
Update Mode - Only the rows that were updated in the Result Table since the last trigger will be written to the external storage (available since Spark 2.1.1). Note that this is different from the Complete Mode in that this mode only outputs the rows that have changed since the last trigger. If the query doesn’t contain aggregations, it will be equivalent to Append mode.
Note that each mode is applicable on certain types of queries. This is discussed in detail later.
>> output mode 는 Complete mode / Append mode / Update 모드 3가지 가 있다.
Complete Mode 는 updated 된 Result Table 이 만들어졌을때졌을때, 외부 저장소에 출력된다.
Append Mode 는 새로운 Rows 들만 Trigger 에 의해 외부저장소에 출력 된다.
Update Mode 란 새롭거나 수정된 Rows 들만 Trigger 에 의해 외부 저장소에 출력 된다. Completed 모드와 다른점은 만약 query 가 Aggregation 을 포함하지 않으면, 이것은 Append Mode 와 똑같이 똑같이 동작한다. (Complete 모드보다 빠를것으로 예상된다.)
To illustrate the use of this model, let’s understand the model in context of the Quick Example above. The first lines
DataFrame is the input table, and the final wordCounts
DataFrame is the result table. Note that the query on streaming lines
DataFrame to generate wordCounts
is exactly the same as it would be a static DataFrame. However, when this query is started, Spark will continuously check for new data from the socket connection. If there is new data, Spark will run an “incremental” query that combines the previous running counts with the new data to compute updated counts, as shown below.
>> 구현하자면 아래와같이 구현한다.
val query = wordCounts.writeStream
.outputMode("complete") or
.outputMode("append") or .outputMode("update")
>> 기존 SQL 과 똑같이 구현하는데, Spark 가 연속적으로 새로운 data 을 체킹한다. (socket 기반) 만약 새로운 Data 가 있으면 Spark 는 증가분에대해서 Query 를 날리게 되고 이전에 돌린 Running count 과 combine 하게 된다. (아래 그림 설명)

Note that Structured Streaming does not materialize the entire table. It reads the latest available data from the streaming data source, processes it incrementally to update the result, and then discards the source data. It only keeps around the minimal intermediate state data as required to update the result
>> % Struct Streaming 은 전체 Table 을 구체화 하지 않는다. 그것은 가장 마지막 사용가느한 data 를 읽고 점진적으로 Result 를 update하는 구조이다.
그리고 원본 source data 는 지우게된다. 이것은 가능한 가장 작은 data 를 유지하는데 그것을 state Data 라한다. Result 를 업데이트하기위한 것이 state Data 이기도 하다.
Basic Operations - Selection, Projection, Aggregation
Most of the common operations on DataFrame/Dataset are supported for streaming. The few operations that are not supported are discussed laterin this section.
>> 기본적인 select 등의 Query 를 아래와 같이 지원한다. 다만 몇 몇개는 지원하지 않는다.
case class DeviceData(device: String, deviceType: String, signal: Double, time: DateTime)
val df: DataFrame = ...
val ds: Dataset[DeviceData] = df.as[DeviceData]
df.select( "device" ).where( "signal > 10" )
ds.filter(_.signal > 10 ).map(_.device)
df.groupBy( "deviceType" ).count()
import org.apache.spark.sql.expressions.scalalang.typed
ds.groupByKey(_.deviceType).agg(typed.avg(_.signal))
Note, you can identify whether a DataFrame/Dataset has streaming data or not by using df.isStreaming.
|
Schema inference and partition of streaming DataFrames/Datasets
By default, Structured Streaming from file based sources requires you to specify the schema, rather than rely on Spark to infer it automatically. This restriction ensures a consistent schema will be used for the streaming query, even in the case of failures. For ad-hoc use cases, you can reenable schema inference by setting spark.sql.streaming.schemaInference
to true
.
Partition discovery does occur when subdirectories that are named /key=value/
are present and listing will automatically recurse into these directories. If these columns appear in the user provided schema, they will be filled in by Spark based on the path of the file being read. The directories that make up the partitioning scheme must be present when the query starts and must remain static. For example, it is okay to add /data/year=2016/
when /data/year=2015/
was present, but it is invalid to change the partitioning column (i.e. by creating the directory /data/date=2016-04-17/
).
>> default 로 Struct Streaming 은 input schema 를 제시하여 입력 받도록 한다. (CSV 의 경우 지원하는데 JSON 은?? kafka 는? )
Basic Operation (like dataframe)
case class DeviceData(device: String, deviceType: String, signal: Double, time: DateTime)
val df: DataFrame = ... // streaming DataFrame with IOT device data with schema { device: string, deviceType: string, signal: double, time: string }
val ds: Dataset[DeviceData] = df.as[DeviceData] // streaming Dataset with IOT device data
// Select the devices which have signal more than 10
df.select("device").where("signal > 10") // using untyped APIs
ds.filter(_.signal > 10).map(_.device) // using typed APIs
// Running count of the number of updates for each device type
df.groupBy("deviceType").count() // using untyped API
// Running average signal for each device type
import org.apache.spark.sql.expressions.scalalang.typed
ds.groupByKey(_.deviceType).agg(typed.avg(_.signal)) // using typed API
2.3.0 부터 지원된다.,
(IPC - 2.2.0 , EPC 2.3.0)
Support matrix for joins in streaming queries
Left Input | Right Input | Join Type |
|
---|
Static | Static | All types | Supported, since its not on streaming data even though it can be present in a streaming query |
Stream | Static | Inner | Supported, not stateful |
Left Outer | Supported, not stateful |
Right Outer | Not supported |
Full Outer | Not supported |
Static | Stream | Inner | Supported, not stateful |
Left Outer | Not supported |
Right Outer | Supported, not stateful |
Full Outer | Not supported |
Stream | Stream | Inner | Supported, optionally specify watermark on both sides + time constraints for state cleanup |
Left Outer | Conditionally supported, must specify watermark on right + time constraints for correct results, optionally specify watermark on left for all state cleanup |
Right Outer | Conditionally supported, must specify watermark on left + time constraints for correct results, optionally specify watermark on right for all state cleanup |
Full Outer | Not supported |
import org.apache.spark.sql.functions.expr
val impressions = spark.readStream. ...
val clicks = spark.readStream. ...
val impressionsWithWatermark = impressions.withWatermark( "impressionTime" , "2 hours" )
val clicksWithWatermark = clicks.withWatermark( "clickTime" , "3 hours" )
impressionsWithWatermark.join(
clicksWithWatermark,
expr( "" "
clickAdId = impressionAdId AND
clickTime >= impressionTime AND
clickTime <= impressionTime + interval 1 hour
"" ")
)
impressionsWithWatermark.join(
clicksWithWatermark,
expr( "" "
clickAdId = impressionAdId AND
clickTime >= impressionTime AND
clickTime <= impressionTime + interval 1 hour
"" "),
joinType = "leftOuter"
)
|
정리하자면, STREAM - STREAM JOIN 에서 INNER 조인은 watermark 가 옵셔날이지만 의미적으로도 써주는것이 좋고 성능도개선된다.
OUTER 조인은 Watermark 가 필수인데 ,그이유는 마지막까지 조인할 대상을 찾고 기다리기 때문에, 만약 null 일 경우 delay time 은 최대가된다.
Joins can be cascaded, that is, you can do df1.join(df2, ...).join(df3, ...).join(df4, ....)
.
As of Spark 2.3, you can use joins only when the query is in Append output mode. Other output modes are not yet supported.
As of Spark 2.3, you cannot use other non-map-like operations before joins. Here are a few examples of what cannot be used.
3. Unsupported!
There are a few DataFrame/Dataset operations that are not supported with streaming DataFrames/Datasets. Some of them are as follows.
In addition, there are some Dataset methods that will not work on streaming Datasets. They are actions that will immediately run queries and return results, which does not make sense on a streaming Dataset. Rather, those functionalities can be done by explicitly starting a streaming query (see the next section regarding that).
Some sinks are not fault-tolerant because they do not guarantee persistence of the output and are meant for debugging purposes only. See the earlier section on fault-tolerance semantics. Here are the details of all the sinks in Spark.