메인 플로우는 여기를 따라한다.https://github.com/facebookresearch/pytorch3d/blob/main/INSTALL.md
참고하여 pytorch3d 개발환경 설치
1. cuda(권장) : 11.7 설치
2. 11.7 에 맞는 cudnn lib 설치
3. pytorch 설치 (2.0.1) with cuda 11.7 torch 버전은 크게 영향은 없는듯
4. cub 환경 설치
cuda\inculde\thrust\cuda\version.h
에 맨 윗출을 추가해놓는다. (#define THRUST_IGNORE_CUB_VERSION_CHECK true)
cuda 11.7 의 경우 cub 11.5 를 포함시켜놓았는데, 이 cub 사용시 pytorch3d 빌드시 문제가된다.
#define THRUST_IGNORE_CUB_VERSION_CHECK true
#ifndef THRUST_IGNORE_CUB_VERSION_CHECK
#include <thrust/version.h>
#if THRUST_VERSION != CUB_VERSION
#error The version of CUB in your include path is not compatible with this release of Thrust. CUB is now included in the CUDA Toolkit, so you no longer need to use your own checkout of CUB. Define THRUST_IGNORE_CUB_VERSION_CHECK to ignore this.
#endif
// Make sure the CUB namespace has been declared using the modern macros:
CUB_NAMESPACE_BEGIN
CUB_NAMESPACE_END
5.내장된 cub 11.5 를 11.7로 바꾸는 작업을 할것이다.
방법은 2가지가 있는데, cuda 설치경로에 cub 11.7 을 덮어씌우는 방법과 cub_home 환경변수 등록후
directory 로 빌드시 포함시키는방법
cub 는 아래서 다운받는다.
https://github.com/NVIDIA/cub/releases
Releases · NVIDIA/cub
Cooperative primitives for CUDA C++. Contribute to NVIDIA/cub development by creating an account on GitHub.
github.com
C:\Program Files\NVIDIA GPU Computing Toolkit\CUDA\v11.7\include\cub
로들어가 기존 cub 파일들 빽업시켜놓고 11.7로 바꿔치기 하였다.
6.
git clone https://github.com/facebookresearch/pytorch3d.git
cd pytorch3d && pip install -e .
를 이용하여 build 성공!
conda 를 이용해서 개발환경 잘 저장해놓자!
7. pytorch 3d 를 이용하여 간단한 gif 생성
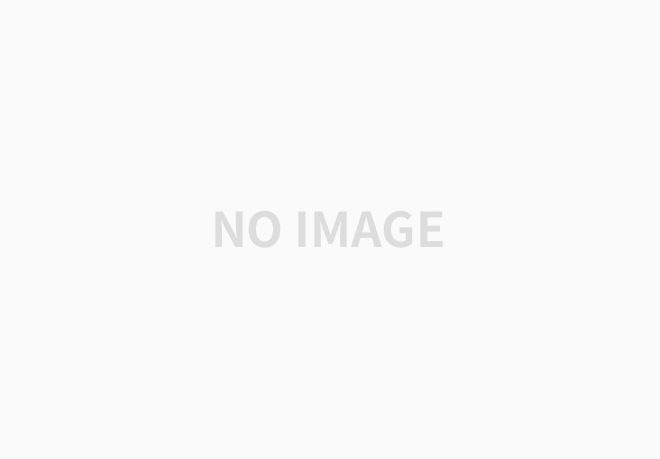